Understanding Machine Learning: From Theory to Algorithms

In the ever-evolving tapestry of technology, machine learning stands as a transformative force, reshaping industries and empowering us to solve complex problems with unprecedented efficiency. This captivating field blends computer science, statistics, and mathematics to create algorithms that can learn from data, identify patterns, and make informed predictions.
4.3 out of 5
Language | : | English |
File size | : | 10280 KB |
Text-to-Speech | : | Enabled |
Screen Reader | : | Supported |
Enhanced typesetting | : | Enabled |
Print length | : | 415 pages |
To fully grasp the essence of machine learning, it is imperative to embark on an intellectual expedition that encompasses both its theoretical underpinnings and the practical arsenal of algorithms it employs. This comprehensive guide will illuminate the intricacies of machine learning, empowering you with a profound understanding of its concepts, techniques, and applications.
Theoretical Foundations: A Cornerstone of Comprehension
The theoretical framework of machine learning provides a solid foundation upon which its algorithms are built. These foundational principles lay the groundwork for understanding the capabilities and limitations of machine learning models.
The Essence of Learning
Machine learning algorithms are designed to learn from data, extracting knowledge and insights that can be applied to new, unseen data. This learning process can be categorized into two primary types:
- Supervised Learning: In this paradigm, the algorithm is presented with a dataset that includes both input features and corresponding output labels. The algorithm learns the relationship between the input and output by studying these labeled examples.
- Unsupervised Learning: Unlike supervised learning, unsupervised algorithms operate on unlabeled data, where no output labels are provided. The algorithm's task is to discover hidden patterns, structures, or relationships within the data.
The Power of Generalization
A crucial aspect of machine learning is its ability to generalize, allowing it to make accurate predictions on new data that it has never encountered during the training phase. This generalization capability is essential for real-world applications, as it enables models to perform effectively on unseen data.
Model Assessment: Measuring Success
Evaluating the performance of machine learning models is paramount to ensure their effectiveness and reliability. Various metrics are employed to assess a model's accuracy, such as:
- Accuracy: The proportion of correctly predicted outcomes.
- Precision: The proportion of true positives among all positive predictions.
- Recall: The proportion of true positives among all actual positives.
Unveiling the Treasure Trove of Machine Learning Algorithms
The practical realm of machine learning is embodied by a vast collection of algorithms, each tailored to specific tasks and data types. These algorithms provide the means to implement the theoretical concepts discussed earlier.
Supervised Learning Algorithms
Supervised learning algorithms excel in tasks where labeled data is available. Some of the most widely used supervised learning algorithms include:
- Linear Regression: A simple yet powerful algorithm for predicting continuous values based on linear relationships.
- Logistic Regression: A specialized algorithm designed for binary classification tasks, predicting probabilities of events.
- Support Vector Machines (SVMs): A versatile algorithm that can handle both classification and regression tasks, known for its robust performance and ability to handle high-dimensional data.
- Decision Trees: A tree-like structure that recursively splits data based on features, resulting in a set of rules for making predictions.
- Random Forests: An ensemble learning algorithm that combines multiple decision trees to enhance accuracy and robustness.
Unsupervised Learning Algorithms
Unsupervised learning algorithms shine in situations where data lacks labels. Prominent unsupervised learning algorithms include:
- k-Means Clustering: An algorithm that groups similar data points into clusters, identifying underlying patterns.
- Principal Component Analysis (PCA): A technique for dimensionality reduction, transforming high-dimensional data into a lower-dimensional space while preserving its key features.
- Anomaly Detection: Algorithms that identify data points that deviate significantly from the norm, flagging potential outliers or anomalies.
Deep Learning Algorithms: Unlocking the Power of Neural Networks
Deep learning, a subset of machine learning, has revolutionized the field with its ability to learn complex patterns from vast amounts of data. Deep learning algorithms are inspired by the structure and function of the human brain, consisting of layers of interconnected artificial neurons.
- Convolutional Neural Networks (CNNs): Specialized deep learning algorithms designed for image and video analysis, recognizing patterns and extracting features.
- Recurrent Neural Networks (RNNs): Deep learning algorithms tailored for sequential data, such as text and time series, capturing temporal dependencies.
- Transformer Networks: A recent advancement in deep learning, demonstrating exceptional performance in natural language processing tasks.
Embarking on the Machine Learning Odyssey
Understanding machine learning requires a harmonious blend of theoretical knowledge and hands-on experience. To truly master this field, consider these practical steps:
- Choose a Programming Language: Python and R are widely used programming languages for machine learning, offering a rich ecosystem of libraries and tools.
- Explore Open-Source Libraries: Libraries like Scikit-learn, TensorFlow, and Keras provide pre-built algorithms and functions, simplifying the development process.
- Practice with Real-World Datasets: Engage in hands-on projects using real-world datasets, gaining practical insights and honing your skills.
- Participate in Online Communities: Join online forums and communities dedicated to machine learning, connecting with experts and learning from their experiences.
: Unveiling the Potential of Machine Learning
Machine learning has emerged as a transformative force, empowering us to solve complex problems and make informed decisions in countless domains. By delving into the theoretical foundations and embracing the practical arsenal of algorithms, we can unlock the full potential of machine learning and contribute to its ever-evolving landscape.
As the realm of machine learning continues to expand, new algorithms and techniques are constantly emerging, promising even greater possibilities. Embrace the excitement of discovery and embark on a lifelong journey of learning and innovation in this captivating field.
4.3 out of 5
Language | : | English |
File size | : | 10280 KB |
Text-to-Speech | : | Enabled |
Screen Reader | : | Supported |
Enhanced typesetting | : | Enabled |
Print length | : | 415 pages |
Do you want to contribute by writing guest posts on this blog?
Please contact us and send us a resume of previous articles that you have written.
Novel
Page
Chapter
Text
Genre
Reader
Paperback
E-book
Newspaper
Paragraph
Preface
Synopsis
Annotation
Footnote
Manuscript
Codex
Classics
Narrative
Biography
Autobiography
Memoir
Encyclopedia
Dictionary
Narrator
Character
Card Catalog
Borrowing
Stacks
Periodicals
Research
Scholarly
Reserve
Academic
Journals
Special Collections
Interlibrary
Dissertation
Storytelling
Reading List
Theory
Namrata Patel
Chris Sharpe
Faizaan Ahmed
Burt Korall
Sara Leman
Kevin Snelgrove
Brent L Sterling
Frost Kay
Peter D Schiff
K P Fen
Darel Ison
Bea Brock
Marcia Stein
Elle James
Fred Bolder
Victoria Villa
Jenny Oldfield
L Q Murphy
Julia Blackburn
David Damschroder
Light bulbAdvertise smarter! Our strategic ad space ensures maximum exposure. Reserve your spot today!
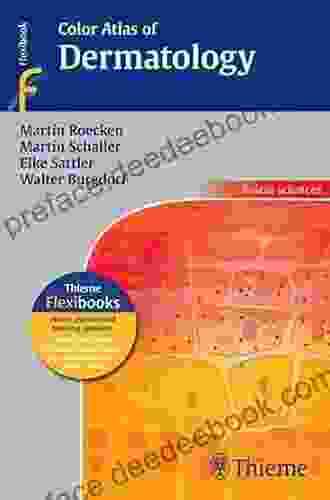

- Jeffery BellFollow ·8.1k
- Zadie SmithFollow ·13.6k
- Rodney ParkerFollow ·5.8k
- Damon HayesFollow ·17.8k
- Jarrett BlairFollow ·3.8k
- Anton ChekhovFollow ·3.8k
- Jeffrey CoxFollow ·10.4k
- Dawson ReedFollow ·2.9k
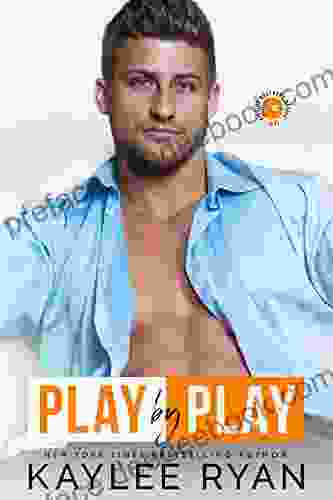

The Legendary Riggins Brothers: Play-by-Play of a...
The Unforgettable Trio: The...
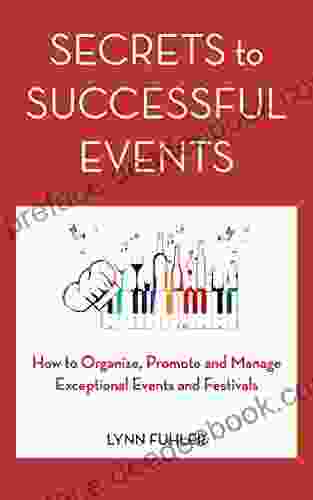

The Ultimate Guide to Organizing, Promoting, and Managing...
Events and festivals have become an...
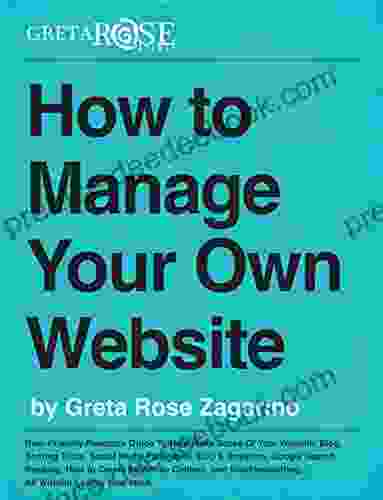

The Ultimate Guide to Managing Your Own Website: A...
In today's digital age, a website is an...
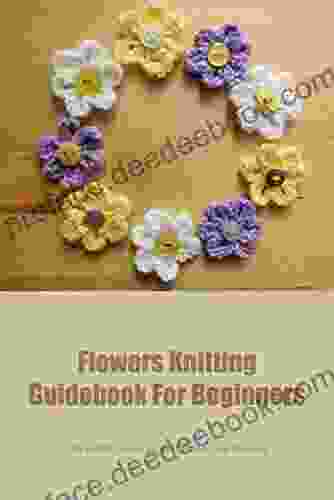

The Detail Guide to Knit Flower for Newbie
Knitting flowers is a...
4.3 out of 5
Language | : | English |
File size | : | 10280 KB |
Text-to-Speech | : | Enabled |
Screen Reader | : | Supported |
Enhanced typesetting | : | Enabled |
Print length | : | 415 pages |